The industrial sector is currently undergoing a triple revolution: digital, environmental and societal. A change that can be explained particularly by the multiplication of sensors on industrial sites, a marked enthusiasm for renewable energy, knowledge of our impact on the environment, and possible energy savings.
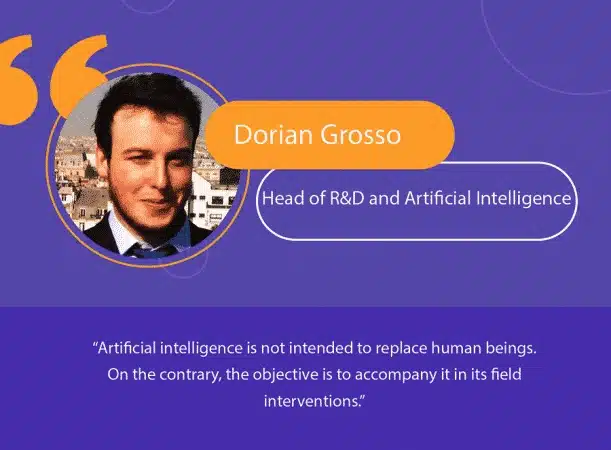
Dorian Grosso is the former head of artificial intelligence (AI) R&D at METRON, and his main mission was to lift the technological barriers linked to AI. We asked him about the role that artificial intelligence can play in research for energy performance at an industrial level. How does it interact with humans in decision-making? What are the special features of the METRON solution?
How do we define artificial intelligence?
In concrete terms, artificial intelligence is committed to developing mathematical techniques and algorithms to give machines the capacity to reproduce - in certain aspects - real intelligence. This standard definition echoes Alan Turing's vision of artificial intelligence in the 1950s. After the test invented by the famous mathematician, artificial intelligence reached its peak when a human would no longer know whether he/she was interacting with a person or a machine.
By looking at this more closely, we discovered two large types of artificial intelligence.
- Symbolic artificial intelligence is generally materialized in the form of a predefined decision tree. The final decision results from a succession of formal answers to questions structured according to logical rules. In fact, symbolic AI is deterministic and can be easily explained. This type of artificial intelligence is also known as the expert system and has been widely used in industry for simple decision making. For example, if the temperature inside an oven is lower than 1500°C, then the fuel flow at one of the burners must be increased.
- Connectionist AI, named in reference to neural networks, is based on algorithms capable of integrating numerous parameters and learning scenarios from empirical examples (learning phase). This type of AI is becoming increasingly popular nowadays. Increasing the processing power of computers, the ability to collect substantial volumes, flows and varieties of data, have enabled us to converge deep neural networks, i.e. to train them in an efficient manner, so that they can correctly carry out a task for which they were trained on new examples never seen before during the learning phase. Such algorithms can be used at several levels within the process to achieve energy optimizations.
Schema of symbolic AI (left). Schema of connectionist AI (right).
What are the limits of AI?
Many people lend AI ill-considered abilities. Even if considerable achievements have been carried out in recent years, a certain number of technological and fundamental barriers must be lifted.
Symbolic AI is easily explained however, it is relatively rigid and can quickly be proven to be inefficient when faced with complex problems. On the contrary, connectionist AI allows complicated tasks to be resolved and can thus be applied to the statistical modelling of industrial processes, the modelling of cost functions, the prediction of electricity consumption, etc. However, in certain cases, the probabilistic results of connectionist AI can be difficult to interpret.
In more general terms, there are many limits to artificial intelligence, and such limits can originate from many things! They can be:
- external - a lack of data, bad quality data or a bad understanding of the energy issues can lead to inefficient algorithms;
- inherent to the problem being addressed - the number of independent variables describing the problem is too large and the number of empirical examples available to train the algorithm is too small. This is known as the curse of dimensionality;
- social - what is the error rate deemed acceptable by the managers of an industrial site in a case of automatic piloting of a very expensive machine? Trust is at the core of the matter, but the understanding of the issue is also generational. It is necessary to know how to be pedagogical in order to convince and not try to replace the human being. We are in a support approach with a strong energy component.
What are the specificities of the AI developed by METRON?
At METRON, we design hybrid AI by combining the two types of artificial intelligence (symbolic and connectionist). This innovative approach, combining ontology and machine learning, is orchestrated in three stages.
Ontology, in short
Ontology is a structured knowledge base that contains data models representing concepts as well as the relationships between these concepts.
Within METRON ontology, we re-transcribe human knowledge of the industrial world. For example, we define all the utilities (compressor, chiller etc.), the actions carried out by the utilities (compressing a fluid, etc.) or the physical properties associated with energetic fluids (pressure, degree of humidity, etc.). One of the objectives of ontology is to define the set of concepts generically only once. The concepts are then reused from one project to the next, which notably grows the scalability of our solution.
We can also assemble concepts defined within ontology, like Lego, in order to build the digital twin of industrial sites. Therefore, we digitalize:
- the configuration of the industrial sites, i.e. the way in which machines are interconnected via energy networks of which the flow is directed;
- the business meaning of the data from the sensors. The time series of the sensors are linked to the business properties stored in ontology;
- the knowledge of machine manufacturers. Key data of technical files can be stored in ontology.
Moreover, ontology is provided with a "semantic reasoner” or "inference machine." We have several types of inferences, including inference via logical rules. For example:
- if we connect an electricity network to a utility, then the utility automatically inherits the property of "electrical power consumed";
- if a sensor measures an amount of energy, then we can automatically calculate the equivalent power, etc.
Then we instantly spread a group of business rules across the digital twin.
Following these two steps, we identify and suggest a set of parameters that can be optimized on the industrial site. It is only then that the algorithms of machine learning come into play. The digital twin allows us to contextualize the data, which is a crucial point. The algorithms then become independent, they are “aware” of the environment in which they operate and are able to access the data that they need easily.
We can then predict the electricity consumption of the equipment, model the utilities and process to establish references and cost functions, to detect excesses, etc. Purpose: be able to minimize the cost functions associated to the CO2 emissions, to the energy costs or even the psychological impact, all while satisfying the business constraints (physical constraints, quality constraints and constraints on the volume of production, etc.).
How does METRON use its AI to increase the abilities of the Energy Manager?
You must understand that AI is not intended to replace humans. The objective is the opposite of this, to support humans in their field operations. From the on-site operator to the plant manager to the Energy Manager, METRON is looking to provide work to all stakholders so that they can concentrate on their high added-value tasks.
AI algorithms can easily handle large volumes, flows and varieties of data and finally, they can understand in a very comprehensive way, the operation of complex industrial systems, which would take a considerable amount of time for the energy provider in certain situations.
Across our platform, our artificial intelligence machine can quickly perform complex analyses and provide the user with a summary in order to make the best decision at a key moment. We can indicate to the user the best path of the control parameters to be implemented (whether on a glass furnace, a compressed air plant or a chocolate production line), so as to improve the energy performance. The operator is free to implement the recommendations, either manually, or via a real-time control system. We also provide a vision that is both comprehensive (aggregation of sites) and local (as close as possible to the machines), as well as energy flows and performance indexes such as benefits performed.
The METRON artificial intelligence machine and humans therefore complement each other.
The technological solution that METRON proposes makes the most of AI by combining the two types of artificial intelligence: symbolic and connectionist. Symbolic AI allows an ontological base to be obtained based on the human knowledge of the technicians working in the field. It is therefore possible to create a digital twin from the plant. The implementation of machine learning algorithms then completes the system to offer optimizations. Concerning industrial energy performance, AI thus allows simulations to be carried out in a specific context. But it has no intention, in any case of replacing humans, which remains the decision-maker of the actions to be implemented in concrete terms.
Want to learn about how the first producer of sugar in the world used AI to reduce their energy consumption?